Cebra
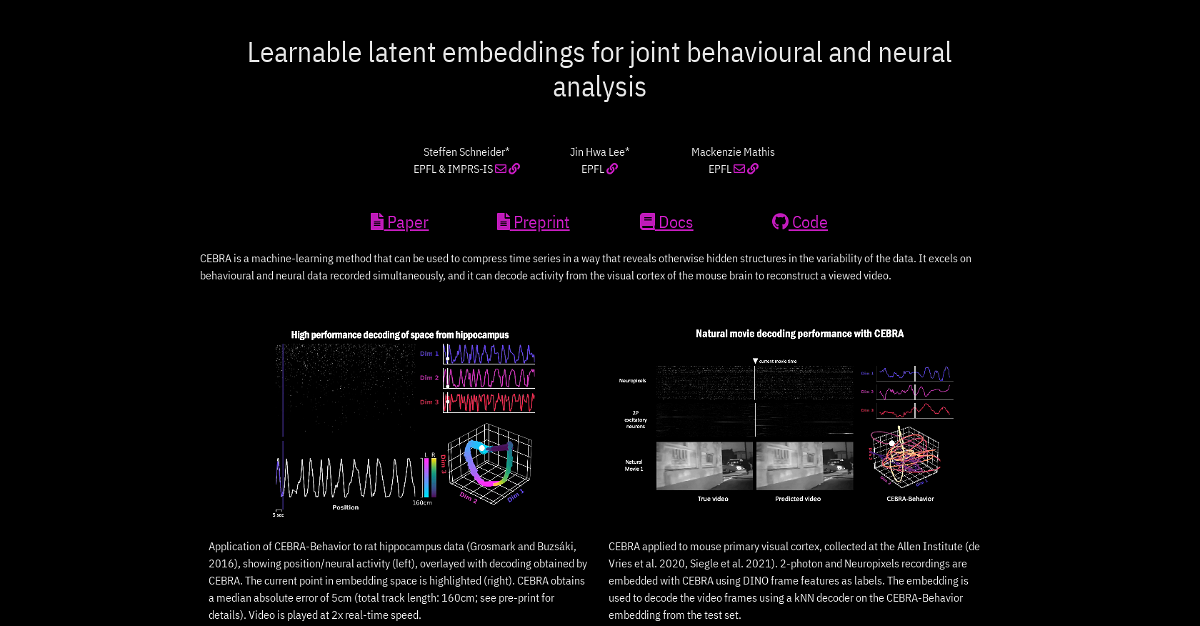
Cebra is a cutting-edge machine learning tool that empowers neuroscientists to unravel hidden patterns within complex behavioral and neural data. This innovative method, developed by researchers at EPFL & IMPRS, excels at compressing time series, revealing intricate structures in data variability. Cebra is particularly adept at analyzing simultaneously recorded behavioral and neural data, enabling the reconstruction of viewed videos from the visual cortex activity of a mouse brain.
Highlights
- Uncovers hidden structures in the variability of data by compressing time series.
- Effectively analyzes simultaneous behavioral and neural data, allowing for the reconstruction of viewed videos from mouse brain activity.
- Offers consistent and high-performance latent spaces for both hypothesis testing and label-free analysis.
Key Features
- Utilizes non-linear techniques to generate consistent and high-performance latent spaces from joint behavioral and neural data.
- Maps behavioral actions to neural activity, providing deeper insights into neural dynamics during adaptive behaviors.
- Creates neural latent embeddings for both hypothesis testing and discovery-driven analysis.
- Validated for accuracy and efficacy across various datasets, including calcium and electrophysiology data, as well as different sensory and motor tasks and behaviors across species.
- Compatible with single or multi-session datasets and can be used label-free.
- Maps and uncovers complex kinematic features, produces consistent latent spaces across diverse data types, and provides rapid and accurate decoding of natural movies from the visual cortex.
Comments
Please log in to post a comment.